Dosimetric Impact of Physician Style Variations in Contouring CTV for Postoperative Prostate Cancer: A Deep Learning–Based Simulation Study
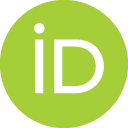
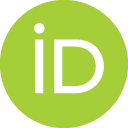
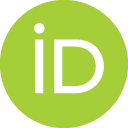
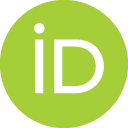
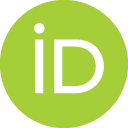
- DOI
- 10.2991/jaims.d.210623.001How to use a DOI?
- Keywords
- Treatment planning; Radiation therapy; Postoperative prostate cancer; Clinical tumor volume; Clinical workflow simulation; Deep learning
- Abstract
Inter-observer variation is a significant problem in clinical target volume (CTV) segmentation in postoperative settings, where there is no gross tumor present. In this scenario, the CTV is not an anatomically established structure, but one determined by the physician based on the clinical guideline used, the preferred trade-off between tumor control and toxicity, their experience and training background, and other factors. This results in high inter-observer variability between physicians. This variability has been considered an issue, but the absence of multiple physician CTV contours for each patient and the significant amount of time required for dose planning based on each physician CTV have made it impractical to study its dosimetric consequences. In this study, we analyze the impact that variations in physician style have on dose to organs-at-risk (OAR) by simulating the clinical workflow via deep learning. For a given patient previously treated by one physician, we use deep learning–based tools to simulate how other physicians would contour the CTV and how the corresponding dose distributions would look for other physicians. To simulate multiple physician styles, we use a previously developed in-house CTV segmentation model that can produce physician style–aware segmentations. The corresponding dose distribution is predicted using another in-house deep learning tool, which, can predict dose within 3% of the prescription dose, on average, on the test data. For every test patient, four different physician style CTVs are considered, and four different dose distributions are analyzed. OAR dose metrics are compared, showing that even though physician style variations result in organs getting different doses, all the important dose metrics except maximum dose point are within the clinically acceptable limit.
- Copyright
- © 2021 The Authors. Published by Atlantis Press B.V.
- Open Access
- This is an open access article distributed under the CC BY-NC 4.0 license (http://creativecommons.org/licenses/by-nc/4.0/).
Download article (PDF)
View full text (HTML)
Cite this article
TY - JOUR AU - Anjali Balagopal AU - Dan Nguyen AU - Maryam Mashayekhi AU - Howard Morgan AU - Aurelie Garant AU - Neil Desai AU - Raquibul Hannan AU - Mu-Han Lin AU - Steve Jiang PY - 2021 DA - 2021/07/11 TI - Dosimetric Impact of Physician Style Variations in Contouring CTV for Postoperative Prostate Cancer: A Deep Learning–Based Simulation Study JO - Journal of Artificial Intelligence for Medical Sciences SP - 85 EP - 96 VL - 2 IS - 1-2 SN - 2666-1470 UR - https://doi.org/10.2991/jaims.d.210623.001 DO - 10.2991/jaims.d.210623.001 ID - Balagopal2021 ER -